The paper by Barker, an assistant professor of mathematics and statistics, appeared in the Online Learning Journal and focused on using topic modeling, a type of computational text mining, to identify participant motivations in enrolling in an online professional development course for teaching statistics.
Assistant Professor of Mathematics and Statistics Heather Barker has published a co-authored paper in the Online Learning Journal (OLJ). OLJ is an open-access journal addressing pedagogy, emerging technology, policy and practice in online environments. This work was a collaboration with co-authors Hollylynne Lee, Shaun Kellogg and Robin Anderson of North Carolina State University.
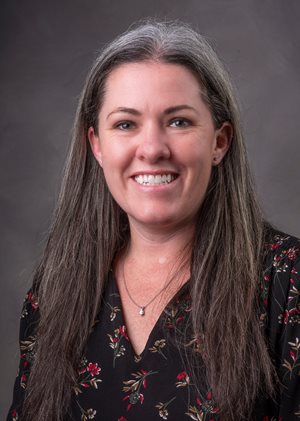
Massive Open Online Courses (MOOCs) have become an increasingly popular way for people to reach their professional development goals. The Friday Institute for Educational Innovation, a part of the College of Education of N.C. State University, developed and hosted MOOC-Eds , free online professional development courses for educators. This research focused on evaluating discussion board forum posts that were made in two different courses developed to enhance teachers’ understanding of statistics and teaching strategies in middle school through introductory-level college courses. The first course was offered seven times and the second course was offered three times, with 3,815 enrolled in all of these sections. The introductory discussion forum posts were analyzed to determine what factors motivated these individuals to enroll in these courses.
Traditionally these sorts of posts would be read and analyzed using qualitative methods, which can be labor-intensive. This study used topic modeling, a computational text mining technique, to see if topic modeling is a viable alternative to traditional qualitative research methods. Three topic modeling methods, using both unsupervised and supervised learning techniques, were applied to these posts. Findings showed one of the unsupervised techniques worked “best” at assigning themes, though the authors encourage anyone attempting this work be sure that the context of the data (in this case the statistics professional development courses) never be separated from the analysis.
Barker, H., Lee, H., Kellogg, S. & Anderson, R.(2024). The viability of topic modeling to identify participant motivations for enrolling in online professional development. Online Learning, 28(1), 175-195. DOI: https://doi.org/10.24059/olj.v28i1.3571