The assistant professor of communication design coauthored a new paper titled “Is this AI trained on Credible Data? The Effects of Labeling Quality and Performance Bias on User Trust.”
Chris (Cheng) Chen, an assistant professor in the Communication Design Department, published a new paper examining data labeling quality, perceived training data credibility, and trust in artificial intelligence under both biased and unbiased AI performance.
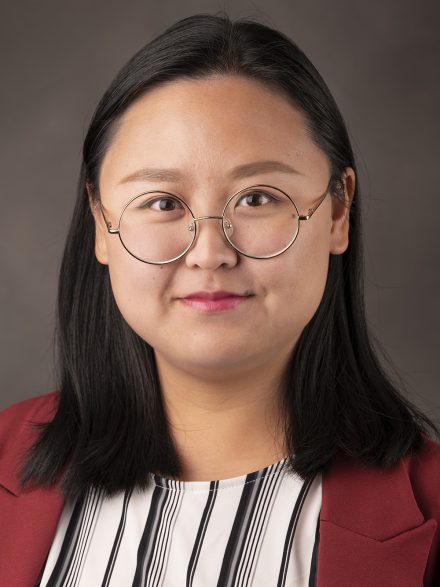
Titled “Is this AI trained on Credible Data? The Effects of Labeling Quality and Performance Bias on User Trust,” the paper was presented by Chen’s coauthor, S. Shyam Sundar of Pennsylvania State University, on April 24 at the 2023 ACM CHI Conference on Human Factors in Computing Systems. The annual event held this spring in Hamburg, Germany, is widely considered the leading international conference of human-computer interaction.
“Given that the nature of training data can cause algorithmic bias, this study asked how best to communicate training data credibility to lay users, so it can help them shape appropriate trust in AI,” Chen said. “By focusing on the accuracy of labeling, we found that showing users the data fed into AI systems was labeled correctly led to higher perceived training data credibility and trust in AI. However, when the system showed signs of being biased, some aspects of their trust go down while others remain at a high level.”
Chen pointed out that supervised machine learning needs to be trained on labeled data, and these data are often labeled by crowd workers, who give pre-defined values, such as happy and unhappy, to each facial image in their example. However, data labeling is highly subjective and often lacks supervision, so labeling accuracy tends to be an issue in labeled data preparation.
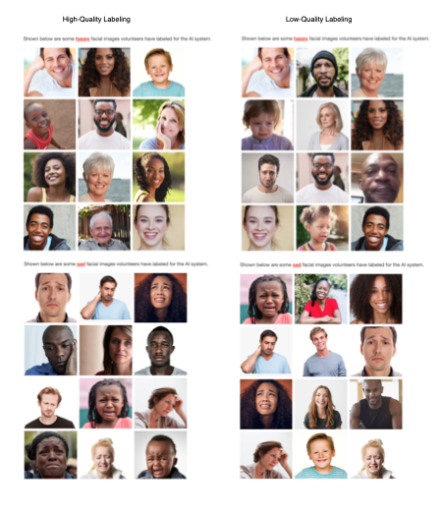
“We created a novel design by showing the labeling practice and a snapshot of labeled data to users before their interaction with the AI system,” Chen said. “Our goal was to see whether users would factor labeling accuracy in when evaluating training data credibility and forming their trust in AI.”
Their findings confirm their original prediction that high-quality labeling results in higher perceived credibility of the training data and trust in AI. But this effect is true only when the AI shows no performance or unbiased performance. In other words, when the AI shows racial bias in facial expression classification, priming users that the training data is credible does not maintain users’ cognitive trust in AI.
“This is a good outcome because it demonstrates that labeling quality can calibrate users’ cognitive trust in AI, matching the level of trust to actual AI performance,” Chen said. “Unexpectedly, users tend to blindly trust the AI system emotionally and behaviorally when they perceive the training data to be credible. This is an old problem in the field of automation, known as automation bias. We hope future studies can come up with novel designs to solve this issue.”
However, their results did vary somewhat from their prediction. How so? “The labeling quality is very persuasive, so involving users in the labeling practice by either asking them to see data labeled by a crowd worker or asking them to participate in data labeling themselves does not add value to the perceived training data credibility,” Chen explained. “Thus, we do not recommend designers to add labeling tasks before users’ interaction with the AI system.”
Chen, a former doctoral student in mass communication at Penn State, has regularly collaborated with Sundar, who co-founded Penn State’s Media Effects Research Laboratory. They coauthored an article in Behaviour & Information Technology looking at why individuals use automated features like autocorrect on iPhone, Smart Reply on Gmail, and autoplay on YouTube. And last summer they also collaborated on an article in Social Media + Society that investigated the habitual and problematic use of Instagram.